The Critical Role of Capturing Feedback in AI Model’s Success
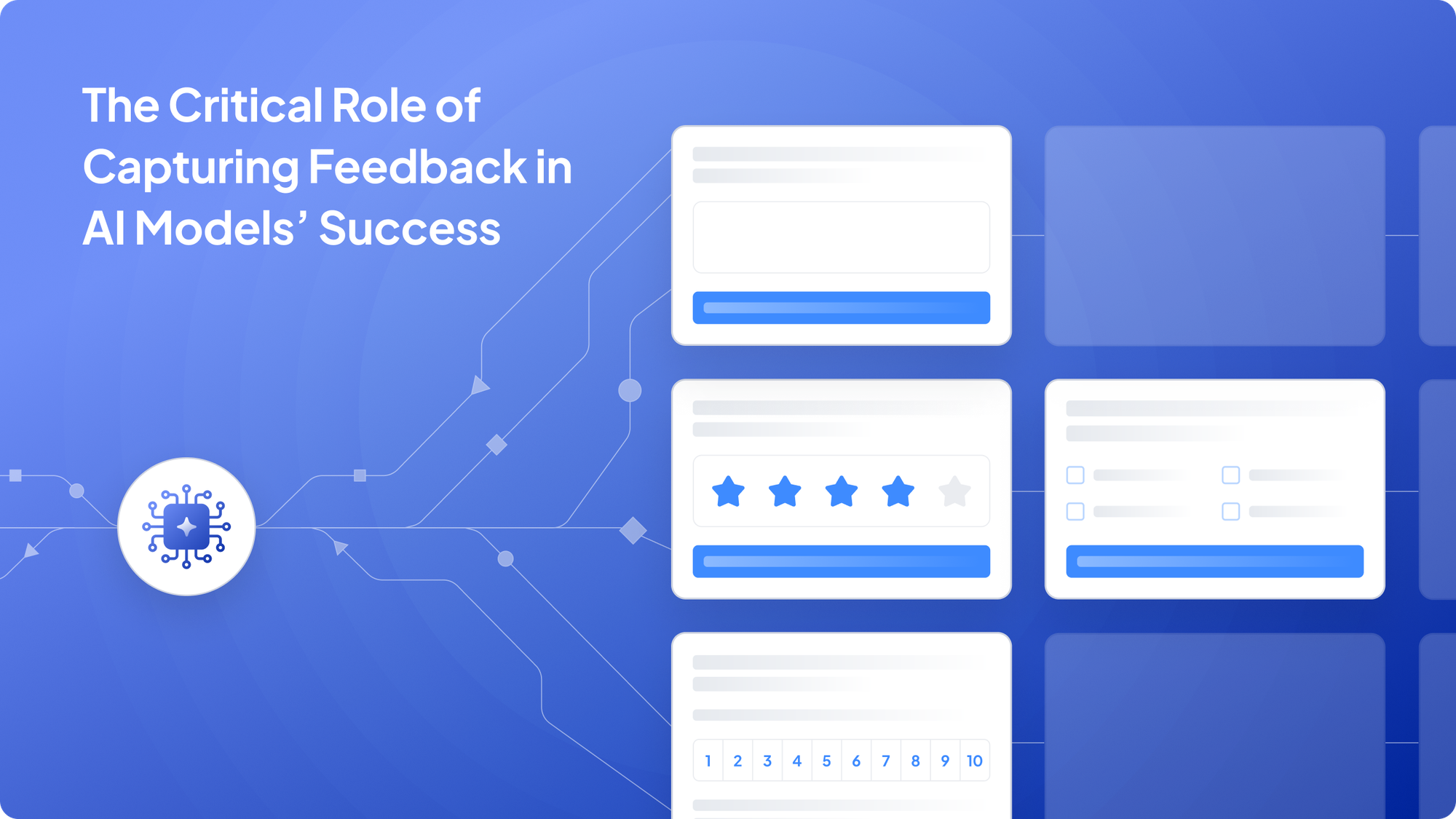
Discussions about the performance of various Gen AI models, relative to other models or previous versions, have become ubiquitous. The assessment appears straightforward for most individuals - they evaluate based on whether the models provide accurate and helpful responses to their queries. However, the evaluation process becomes significantly more complex when deploying AI models at scale in business environments.
For example - Integrating an AI model into a sales CRM system introduces key questions:
- Are sales representatives effectively utilizing the model's insights and recommendations?
- How accurately does the model predict potential leads and sales opportunities?
To answer these questions and drive continuous improvement, capturing and analyzing end-user feedback is essential.
Our previous blog discussed how you can easily integrate AI/ML model insights into business applications using Data Apps. Data Apps allows you to connect to AI/ML sources and build visualizations that business users can easily consume. However, this is only one piece of the puzzle. The true value comes from understanding how the AI/ML models are performing in the real world and getting feedback from the users interacting with them.
Enabling feedback loops is critical to establishing trust in AI-powered business systems. Once you have integrated the AI model results into a business application, you would want to know if users are actively using the model results and how well they are performing.
Challenges in Capturing AI Model Usage & Performance Once They Go Live
Feedback is often collected in an ad-hoc manner, with teams considering it only after a model is live. Feedback is commonly gathered through surveys, but these results are rarely linked back to the model evaluation process. The inconsistency in feedback methods adds to the challenge - different versions of the model may use entirely different surveys with varying questions, making it difficult to compare results and track improvements over time.
This delay hinders the establishment of robust, business-informed iteration cycles that could enhance model performance.
Introducing Human-in-the-Loop Feedback with Data Apps:
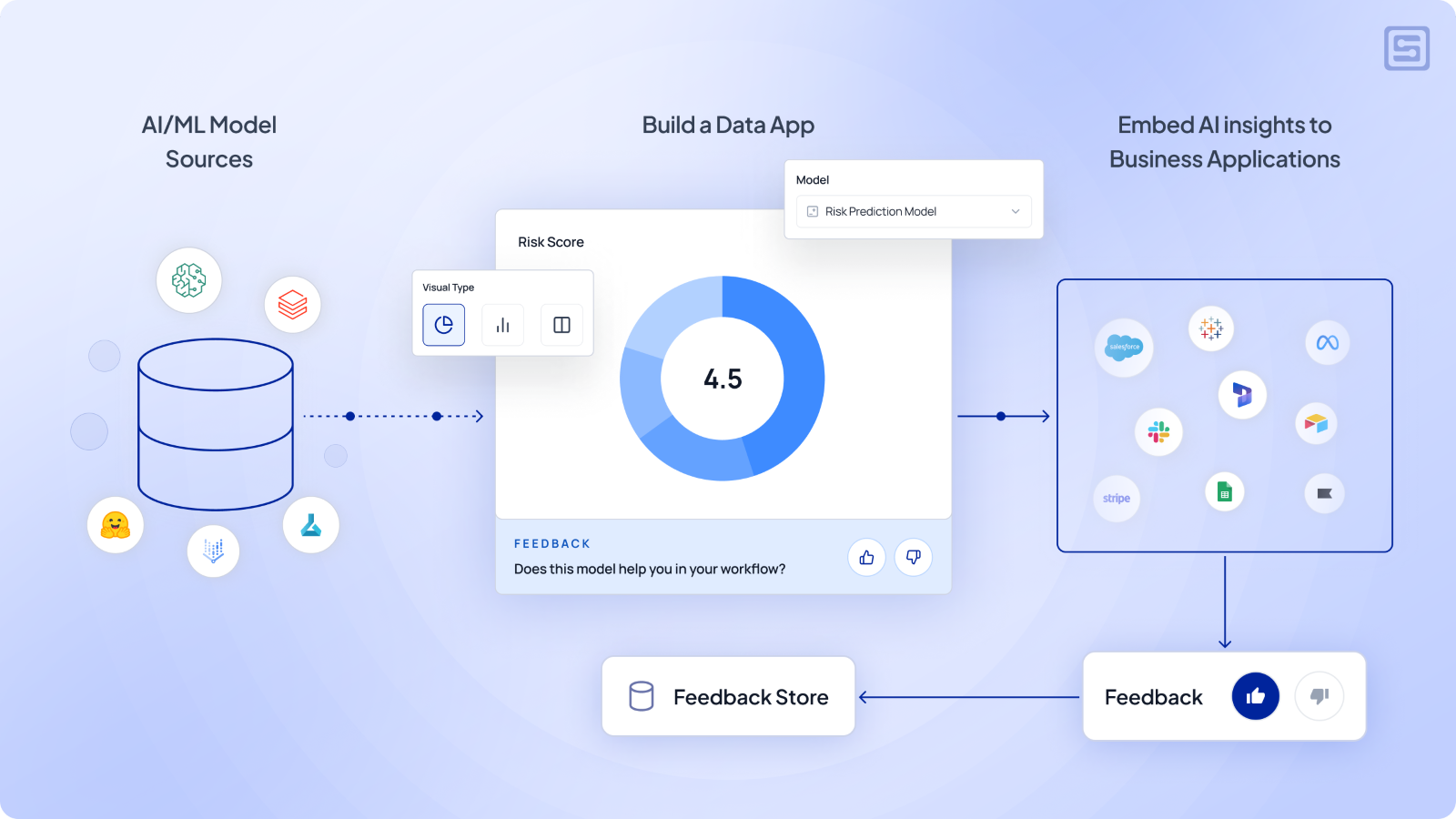
Data Apps bridges this gap by allowing you to configure and capture user feedback when they access the model insights within a business application. There are two ways to collect end-user feedback while creating a Data App:
There are two ways to collect end-user feedback:
- Qualitative feedback: Allows you to capture end users’ observations/experiences with free-form input such as text
- Quantitative feedback: Allows you to capture end users’ feedback in the following ways:
- Thumbs up/down: Allows the end users to give a Yes/No rating as to whether they found AI insights helpful
- Scale-based input: Allows the end user to rate the usefulness on a scale of 1 – n, where n can be configured from 3 to 7
- Single/Multi-select options: Allows the user to select from custom choice options
Measure AI Adoption & Usage with a Dashboard View
Once you have integrated the AI model results into a business application with Data Apps, you can view how actively users are accessing the model results and how well the model results are performing.
The Data Apps Reports section allows you to view the active usage of your AI models deployed within a business application in real-time. This is useful in scenarios where you have two versions of the model results, and you want to test which model insights is preferred by the business users. By looking at the metrics such as total sessions and the feedback captured, you can take a call to roll out the right model to the larger audience.
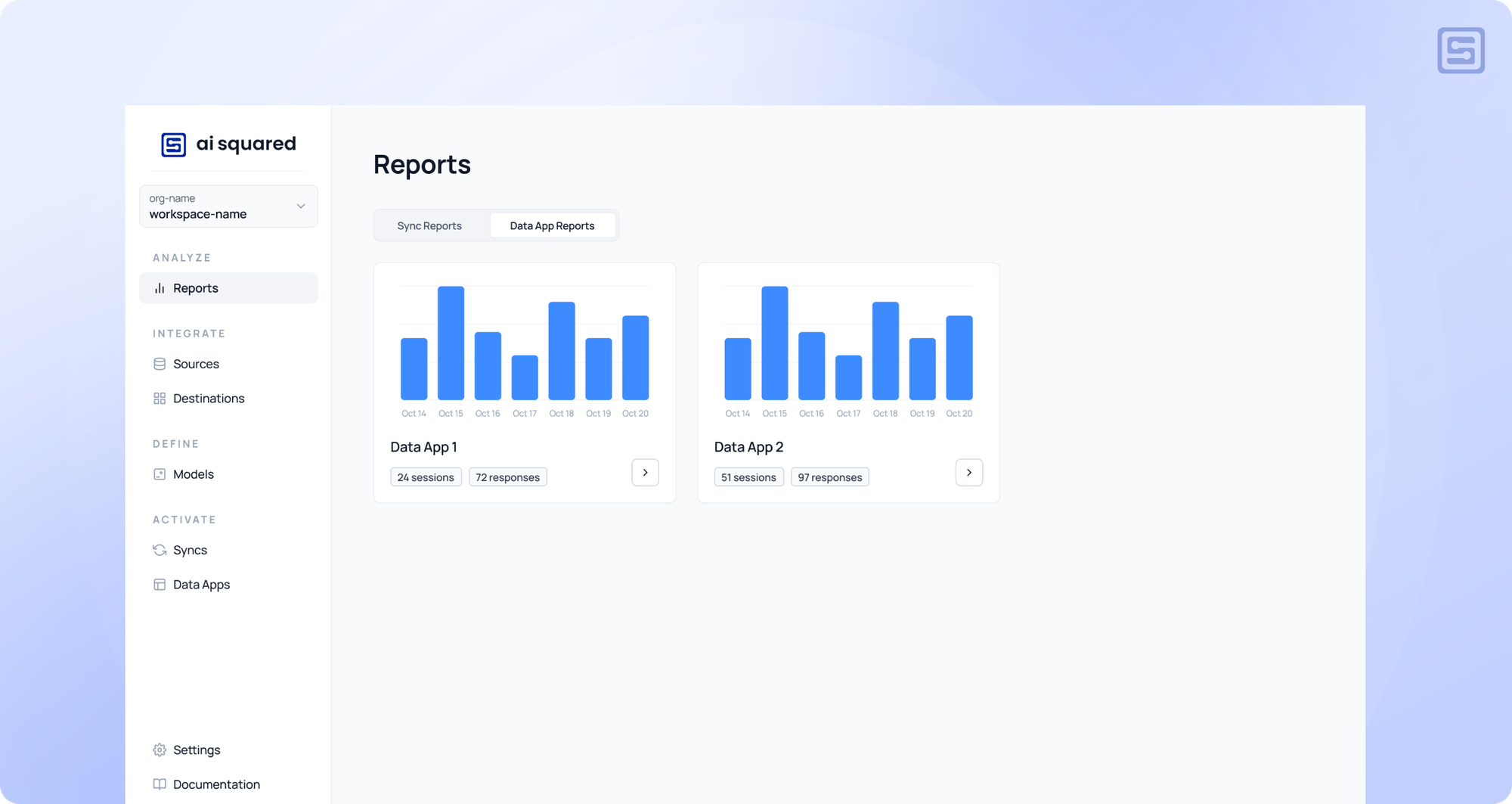
You can also click on a specific Data App and analyze the performance in detail by answering the most pressing questions such as:
- How many employees access your AI model results?
- How actively engage with a Data App by providing feedback?
- How satisfied are they with the model results?
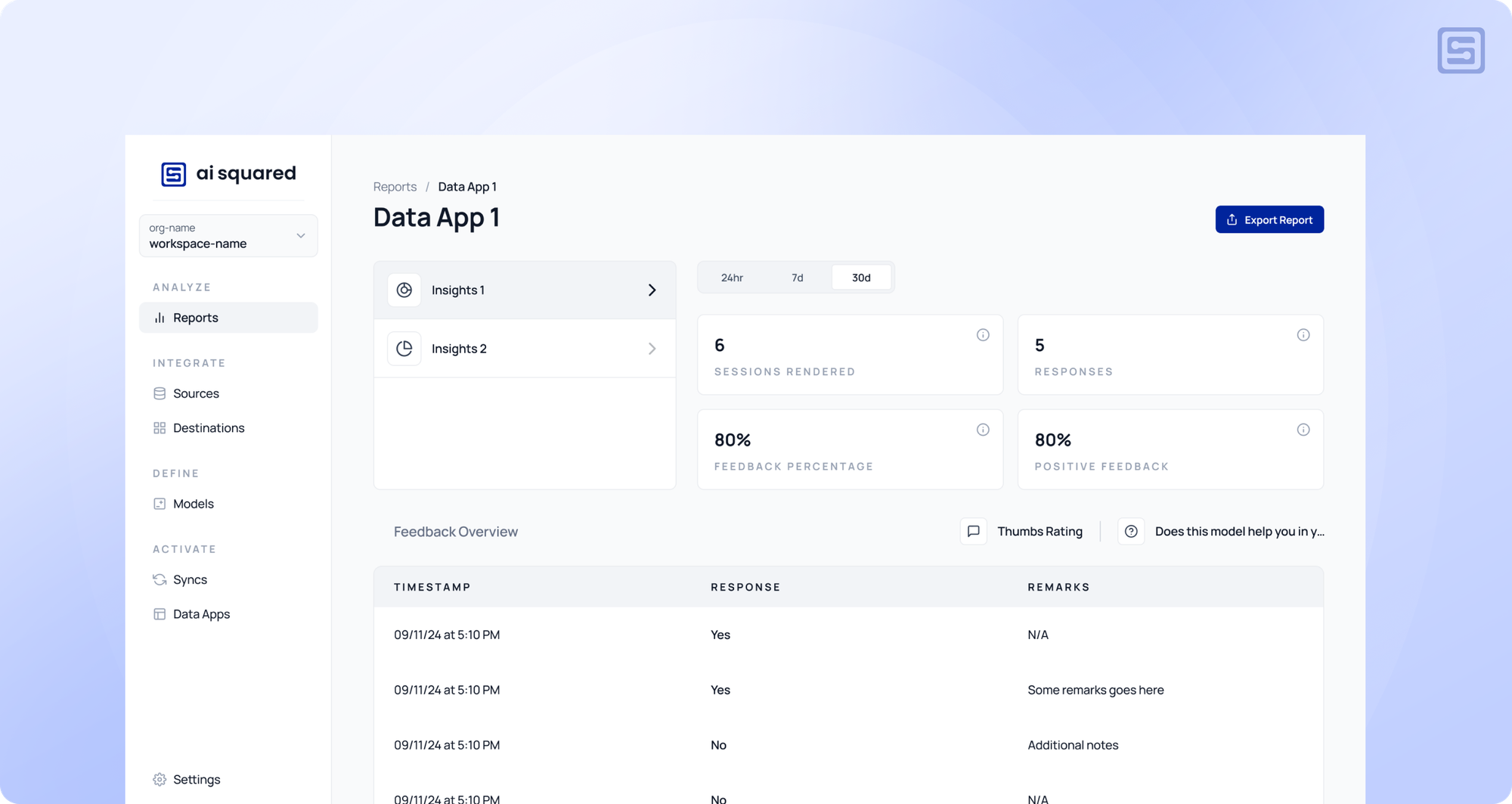
Understand How Well AI Models are Performing
The Feedback Overview section on the Data Apps reports provides a detailed look at individual feedback received.
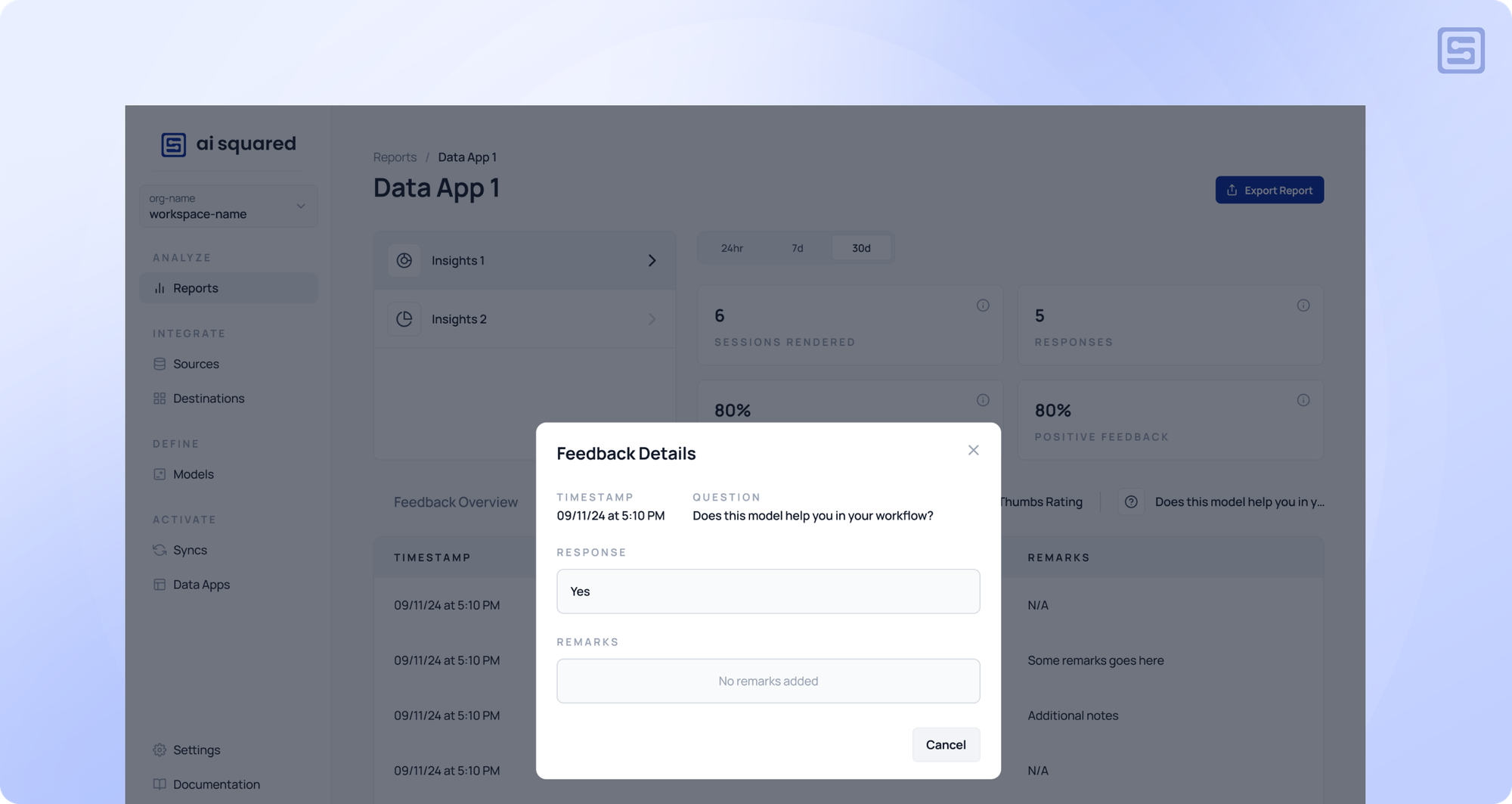
You can download the feedback data at an aggregate level, over a period of time, to further analyze and use it for model enhancements.
Achieving Faster Time-to-Value for AI/ML Models:
Capturing user feedback on AI models provides valuable insights beyond usage frequency. It reveals the perceived value of model outputs and enables rapid, real-time adjustments to performance. This agility allows for quick responses to new information and evolving market dynamics.
Integrating user input into model development bridges the gap between AI capabilities and industry domain knowledge, resulting in more robust and contextually aware systems.
When developing AI-powered products, accelerating the process from prototyping to scaling is crucial. User feedback plays a pivotal role in this journey, significantly impacting the building, deployment, and expansion of AI/ML models.
Data Apps with feedback enabled allows you to not only ship models faster to business teams but also capture continuous feedback on AI insights being delivered directly within business workflows. Schedule a demo to see how you can accelerate the time-to-value of AI/ML models.