Understanding Data Governance and AI in Financial Services
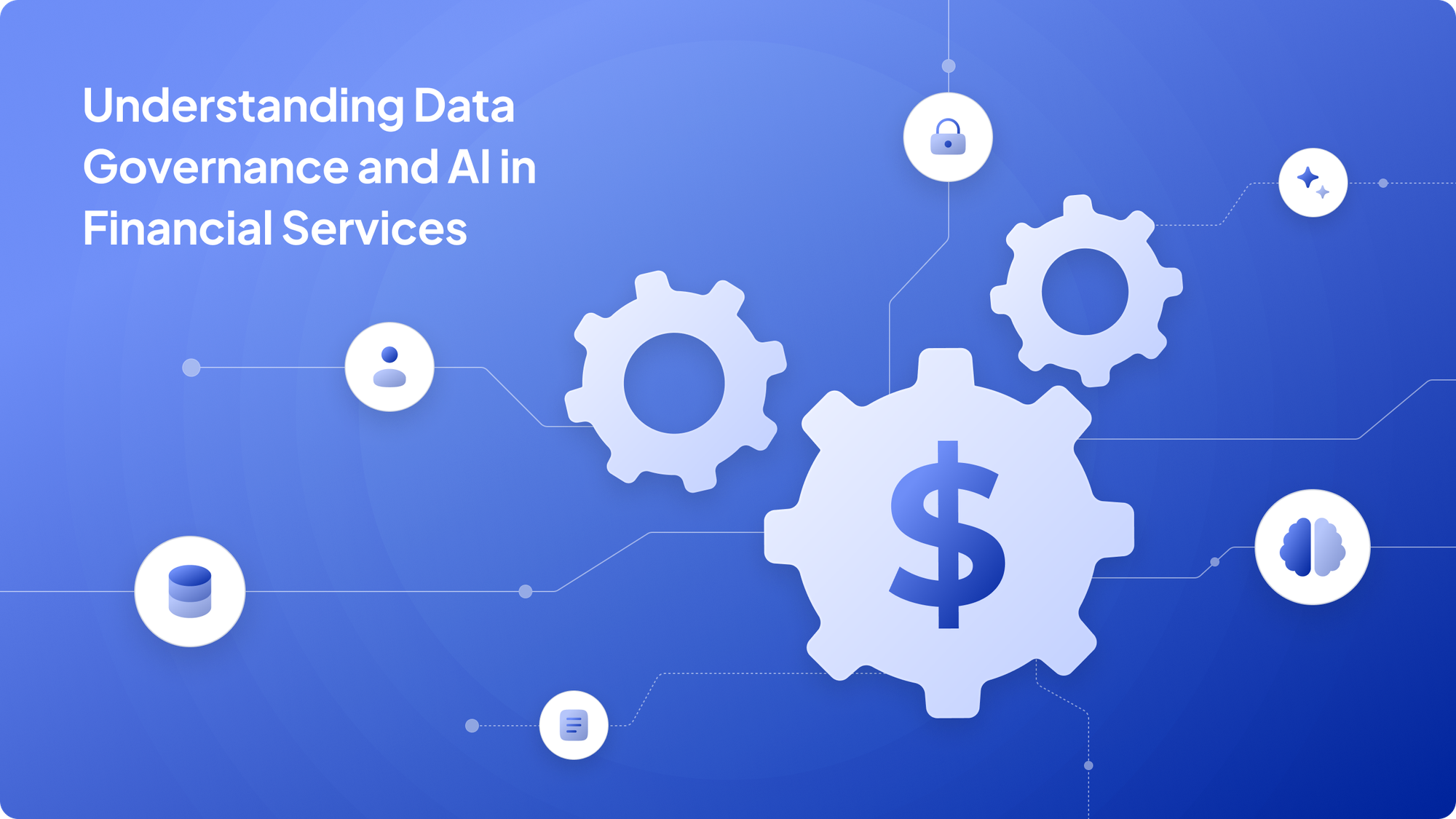
Within banks and other financial services, there are copious amounts of sensitive data being handled. When an individual opens an account, they provide information that needs protection, from name and address to social security number. With this type of data, financial institutions can improve services and make informed decisions – only if the data is used safely.
Data governance involves establishing a continuous process to create and improve policies and standards around managing data to ensure the information is usable, accessible, and protected. At the same time, creating a data governance framework can feel overwhelming, especially in the beginning.
Data governance in financial services is a comprehensive framework for managing, protecting, and utilizing data assets within financial institutions. It ensures data accuracy, security, consistency, and compliance with regulatory requirements.
A few things to keep in mind when creating a data governance framework:
- Data quality management: Ensuring data is accurate, complete, consistent, and reliable throughout its entire lifecycle.
- Security and compliance: Protecting sensitive information and adhering to regulatory requirements while maintaining data integrity.
- Data lifecycle management: Tracking and managing data from creation to deletion, optimizing its value, and minimizing risks.
- Ownership and accountability: Clearly defining responsibilities for data creation, maintenance, and usage across the organization.
- Standardization: Establishing uniform processes, definitions, and formats to enable seamless data integration and understanding.
By prioritizing data governance, financial institutions can transform data from a potential liability into a strategic asset that can drive innovation, enhance customer experience, and support sustainable growth.
The Intersection of Data Governance and AI
The intersection of data governance and AI represents a shift in how organizations in financial services manage and utilize their data. The relationship between the two is "symbiotic." AI is both driving and being driven by data governance. Companies and institutions are building and establishing AI into every component of the business, be it the front office, sales and trading, legal contracts, and/or cost efficiency gains. AI is accelerating the advancement of data management and governance frameworks, as organizations within financial services seek to derive actionable value from AI applications. This value is heavily dependent on the quality and governance of an organization's data infrastructure.
Is AI driving Data Governance? Or vice versa?
The relationship between AI and data governance is particularly critical in financial services. AI enhances financial data governance by automating complex tasks like sensitive financial data classification, regulatory compliance tracking, and risk management metadata management. This automation is especially valuable in the financial sector, where AI-powered tools can discover, catalog, and securely integrate data across multiple sources while maintaining strict compliance with financial regulations like GDPR, Basel III, and industry-specific reporting requirements. Machine learning models can adapt to evolving financial data patterns and governance standards, helping institutions manage increasingly complex regulatory landscapes. Conversely, robust data governance frameworks are crucial for maximizing AI's value in financial services, ensuring responsible, secure, and compliant deployment of advanced technologies that protect sensitive customer and transaction data.
Impact of AI Regulations on Data Governance
AI regulations will develop gradually over the next few years. They will begin with rules for foundation models and major players, then expand to address practical applications across corporations and financial institutions. For the next few years, any individual or groups working in this space cannot and should not rely on the current regulatory landscape. It will have to evolve, and the people and practitioners will have to operate assuming that there will be inevitable changes.
As AI continues to evolve, organizations must adapt their data governance frameworks to meet new challenges and regulatory requirements. With governments implementing stricter rules around data privacy and security, companies are being compelled to update their governance practices to ensure compliance. This often requires investing in more robust technology and providing additional training to promote responsible data handling. The intersection of data governance and AI is creating a more dynamic and efficient approach to managing data assets, establishing a reciprocal relationship: while AI enhances data governance practices, strong data governance remains crucial for the ethical and effective implementation of AI technologies.
The contrast between the new and previous administrations' AI regulation reveals a significant policy shift. While the previous administration implemented comprehensive federal oversight through Executive Order 14110, emphasizing civil rights and safety, the new administration prioritizes deregulation and technological innovation. Upon returning to office in January 2025, the new administration immediately revoked the previous Executive Order, signaling a commitment to reducing government interference in AI development.
The core difference between the previous and current administrations lies in their perspectives: The previous administration focused on careful government oversight and transparency, whereas the current administration prioritizes economic competitiveness and fewer restrictions. This approach means potentially rolling back agency-level AI oversight and creating a more business-friendly environment for technological advancement, while still maintaining U.S. technological leadership in the global market.
Challenges & Opportunities
The massive scale of data required for AI systems in financial institutions presents both significant challenges and opportunities in data management. Financial organizations typically need billions of data points from diverse sources like customer transactions, market histories, risk assessments, and regulatory records for developing and training AI models. At such volumes, maintaining consistent data quality becomes exponentially more difficult, particularly given the complex and sensitive nature of financial data. On the other hand, AI itself offers solutions to these challenges: AI-powered tools can automate data cleansing and validation processes, while also discovering and classifying data across an organization's landscape automatically, significantly reducing the manual effort required for data identification and categorization.
Data silos pose significant challenges for AI implementation in financial institutions while also presenting opportunities for improvement. In financial organizations, these isolated data repositories often separate critical information across departments like risk management, customer service, compliance, and trading, leading to inconsistent data inputs that may cause AI models to generate incorrect or partially correct financial analyses and decisions. Additionally, siloed systems in financial contexts often lack uniform security measures, making them vulnerable to unauthorized inputs and potential prompt injection attacks that could compromise sensitive financial data. However, breaking down these silos offers considerable benefits: it can significantly improve AI model accuracy in financial applications, with the potential to achieve over 90% accuracy in areas like risk assessment and fraud detection, while integrating data sources into a unified system enhances both data accessibility and usability across the financial organization.
Data security in AI systems presents a complex balance of challenges and opportunities within financial institutions. The extensive data collection required for advanced analytics and AI technologies raises significant privacy concerns, particularly around sensitive customer financial information, personal identifiers, and transaction histories that could be exposed to potential breaches. These data requirements increase the risk of unauthorized access to critical financial data, with potential consequences ranging from regulatory penalties to severe reputational damage. Data silos in financial organizations can create additional security vulnerabilities by maintaining inconsistent protection measures across different systems handling customer accounts, transaction records, and regulatory reporting. However, AI technology also offers powerful solutions to these security challenges: it can automate compliance checks and policy enforcement, ensuring consistent application of data governance policies across complex financial ecosystems. Furthermore, advanced AI-powered security measures can provide round-the-clock safeguards against cyber threats, implementing sophisticated anomaly detection and real-time threat monitoring that significantly enhances overall data protection capabilities in financial contexts.
Building a Foundation for AI-Driven Data Governance
Navigating data governance and AI in finance institutions demands a balanced approach - one that embraces innovation while safeguarding sensitive information. Financial organizations need to create an integrated framework that prioritizes data quality, transparency, and ethical use of AI while viewing data governance and AI as complementary strategies for risk management and regulatory compliance. Success in this area can be achieved by investing in automated data profiling for financial risk assessment, implementing explainable AI models that meet regulatory scrutiny, and fostering cross-functional collaboration across compliance, risk, and technology departments. Through these efforts, financial institutions can build a resilient ecosystem that maximizes the potential of AI while maintaining strict compliance and data integrity.
The landscape of data governance and AI in financial services is rapidly evolving, characterized by a convergence of technological innovation, ethical considerations, and increasingly complex regulatory requirements. Financial organizations are increasingly leveraging AI to transform data management, making it more intelligent, transparent, and secure in handling sensitive financial information. The key trends point towards a future where data governance is not just a compliance requirement, but a strategic asset for financial institutions – powered by AI-driven insights, enhanced by cloud technologies, and guided by principles of transparency, fairness, and customer data protection. As financial businesses navigate this complex ecosystem, the ability to balance technological capabilities with ethical considerations and robust governance will be crucial in unlocking the full potential of data and AI while maintaining customer trust and regulatory compliance.